Mitigating Survivorship Bias in Scholarly Research: 10 tips to enhance data integrity
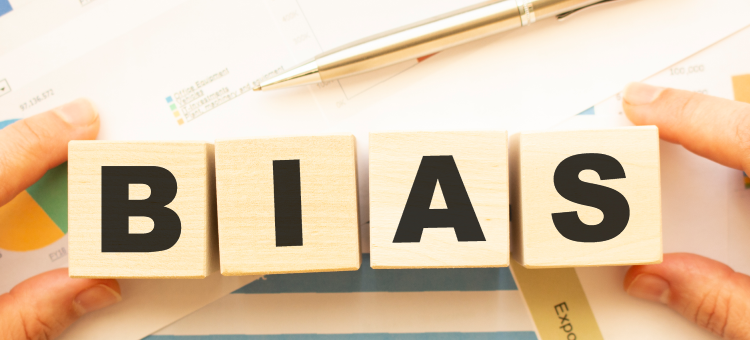
In the realm of research, uncovering of truth requires a vigilant eye and an unwavering commitment to objectivity. But what if our quest for knowledge is tainted by an unseen deception?
Imagine a world where only the winners tell their tales, where failures fade into oblivion. This can happen in research too, with something called survivorship bias. Survivorship bias obscures our vision and distorts our understanding. It tricks us into thinking that success is normal and guaranteed, while hiding important lessons from those who didn’t succeed. This can lead us to false conclusions and misguided decisions. In this thought-provoking article, we explore survivorship bias in research, exploring its far-reaching implications and offering invaluable tips to shield ourselves from its influence and ensure data integrity.
What Is Survivorship Bias?
Survivorship bias is a type of selection bias that occurs when we only consider the “survivors” of a process or event and ignore those who did not survive or were excluded. Survivorship bias derives its name from the error that occurs when a dataset solely focuses on the “surviving” observations, neglecting to account for those that did not endure or survive. This bias arises from the omission of non-surviving elements within the data set, creating an incomplete and skewed representation of reality. This bias can be found in various domains, including business, finance, history, and of course, research. In research, this can happen when we only analyze the data from successful participants or interventions and ignore those who have dropped out or failed. To mitigate survivorship bias, researchers need to be aware of its existence and actively incorporate diverse perspectives and data into their analyses.
The Impact of Survivorship Bias in Research
The risks associated with survivorship bias can lead to a distorted perception of reality, giving rise to incorrect conclusions and decisions. By overlooking the failures or unsuccessful cases, we might underestimate the risks, overestimate the chances of success, and adopt faulty assumptions or strategies. To avoid this bias, it is crucial to look at both the survivors and the non-survivors, and to analyze the whole distribution of outcomes, not just the extreme ones.
Examples of Survivorship Bias in Research
Health and medical research: Survivorship bias can impact medical studies that focus solely on patients who have successfully completed a treatment or intervention. This has been observed particularly in disease diagnoses, specifically when examining post-diagnosis survival rates. This bias arises from the fact that patients with the best prognosis, typically characterized by factors such as younger age, good health history, and higher fitness levels, tend to exhibit higher survival rates compared with those with a less favorable health history. However, the calculation of survival rates often excludes patients with a poorer health history who do not survive, resulting in an overrepresentation of healthier individuals in the data.
In the case of global events like the COVID-19 pandemic, accurately assessing the impact of the virus poses challenges. Epidemiologists and medical professionals caution that relying solely on survival rate calculations does not provide a comprehensive picture. For instance, individuals who die without having been tested for COVID-19 cannot be included in the official death count associated with the virus. This limitation can potentially introduce survivorship bias when analyzing data related to the disease, particularly in countries where testing capacity and infrastructure are overwhelmed, resulting in incomplete data and potential distortions in survival rate calculations.
World War II plane research: During World War II, statistician Abraham Wald and his research team at Columbia University encountered a fascinating example of survivorship bias in their study of bomber planes. Their task was to recommend areas for reinforcement on the aircraft based on an analysis of the damage sustained by returning planes. Initially, they focused on the locations that had suffered the most damage, intending to reinforce those areas to improve survivability.
However, Wald astutely recognized the survivorship bias at play. He realized that they were only considering the planes that had made it back safely, while ignoring the planes that were shot down. This revelation led to a paradigm shift in their approach. Wald understood that the heavily damaged areas on the returning planes were the ones that could withstand such damage and still return. On the other hand, the planes that were shot down likely sustained damage in different areas. Thus, their analysis was skewed by the survivorship bias, as it failed to account for planes that didn’t survive. To overcome this bias, Wald’s team recommended reinforcing the areas on the planes that were underrepresented in the data, specifically, the areas around the motors and cockpit. They realized that the planes hit in these critical areas never made it back, indicating the need for reinforcement in those spots. This breakthrough in their research allowed them to make more accurate predictions and potentially save many lives.
Survivorship bias can have several significant impacts on research, potentially compromising the validity and reliability of findings. Here are some key impacts of survivorship bias on research:
1. Distorted conclusions: Survivorship bias arises from a fundamental misconception of correlation and causation, thus resulting in distorted conclusions and misrepresentations of reality. By focusing only on the surviving or successful outcomes, researchers may draw inaccurate generalizations or make unwarranted claims about the entire population or phenomenon under study.
2. Incomplete understanding: Survivorship bias can result in an incomplete understanding of the subject being researched. By excluding or neglecting non-survivors or failures, researchers miss crucial data points that could provide valuable insights into the underlying mechanisms or factors contributing to outcomes.
3. Biased sample selection: Survivorship bias can impact the selection of research participants or samples. Researchers may unintentionally bias their sample by only including individuals or entities that have survived or succeeded, excluding those that have not. This bias results in skewed outcomes and restricts the applicability of findings to the wider population.
4. Skewed statistical analysis: Survivorship bias can distort statistical analysis by overlooking non-surviving or failed cases. This bias can lead to skewed distributions, overestimation of effect sizes, or misleading statistical relationships. Consequently, the statistical significance and interpretations of results may be inaccurate or misleading.
5. Publication bias: Survivorship bias can also affect the publication of research findings. Journals and publication outlets may be more inclined to publish studies with positive or significant results, while overlooking studies with non-significant or negative findings. This publication bias can create a skewed representation of the research landscape, leading to an overemphasis on successful or positive outcomes.
6. Impaired replication and generalizability: Survivorship bias can hinder the replication of research studies. If only successful or surviving cases are considered, it becomes difficult to replicate the results with the same level of success or generalize the findings to different populations or contexts. This limits the robustness and external validity of research.
7. Misguided policy and decision-making: The impact of survivorship bias on research can extend to policy and decision-making processes. If research findings are biased due to survivorship bias, policy decisions based on these findings may be ineffective, as they fail to consider the full spectrum of outcomes and experiences.
10 Tips to Evade Survivorship Bias
Now that we’re aware of the perils that survivorship bias presents, it’s time to arm ourselves with the knowledge and strategies to evade its clutches. Here are some indispensable tips to help us break free from its deceptive grip:
1. Define the population or sample inclusively: Make sure to clearly define the population or sample from which data is collected, including all relevant cases, not just the successful ones. Avoid excluding or overlooking any subgroups, outliers, or non-responders as they may carry important information.
2. Use random sampling techniques: Random sampling techniques, such as random sampling, stratified sampling, or cluster sampling, can help ensure that each member of the population or sample has an equal chance of being included in the study. This can minimize the risk of selective sampling and survivorship bias.
3. Collect data on non-survivors or non-responders: Collect data on non-survivors or non-responders, including their characteristics, reasons for dropping out or not responding, and any potential impact on the results. This can provide a more complete picture of the phenomenon under study and help identify any biases that may arise from survivorship bias.
4. Include multiple time points: If studying a dynamic phenomenon over time, include multiple time points to capture changes and fluctuations. Avoid only looking at the endpoint or outcome, as this can lead to survivorship bias by ignoring the experiences of those who did not reach the endpoint.
5. Use multiple data sources: Relying on a single data source can increase the risk of survivorship bias. By using multiple data sources, such as different datasets, surveys, or interviews, you can obtain a more comprehensive and diverse perspective, minimizing potential biases.
6. Consider multiple outcomes: Avoid only focusing on a single outcome or measure. Consider multiple outcomes, including both positive and negative outcomes, to capture the full range of possibilities. This can help avoid cherry-picking or emphasizing only the successful outcomes, reducing the impact of survivorship bias.
7. Conduct sensitivity analysis: Conduct sensitivity analysis to test the robustness of the findings to different assumptions or scenarios. This can help identify the potential impact of survivorship bias on the results and provide more accurate and reliable estimation of the phenomenon under study.
8. Challenge assumptions: Be mindful of assumptions made in the research process, such as assuming that the survivors or successful cases are representative of the entire population. Challenge these assumptions and critically evaluate their validity to avoid survivorship bias.
9. Seek diverse perspectives: Seek feedback and input from diverse perspectives, such as colleagues, experts, or participants from different backgrounds. This can help identify potential biases, including survivorship bias, that may be overlooked from a single perspective.
10. Transparently report on limitations: Be transparent and honest about the limitations of your research, including the potential for survivorship bias. Acknowledge any limitations, biases, or challenges in the research process and report them clearly in your findings. This can enhance the credibility and integrity of your research findings.
Conclusion
Survivorship bias is a common but often overlooked bias in research and poses a significant threat to the integrity of research findings. To mitigate the impacts of survivorship bias in research, it is essential for researchers to be aware of its presence and actively take measures to address it. This includes considering non-survivors or failures, broadening the sample selection, transparently reporting limitations, promoting replication studies, and encouraging the publication of diverse research outcomes. Implementing the tips outlined in this article can help escape its clutches and foster a more accurate understanding of the world. By minimizing survivorship bias, researchers can enhance the credibility and applicability of their research findings. Have you encountered instances of bias in your research? How did you address them? We encourage you to share your experiences by writing to us or leaving a comment below. We value your insights and would love to hear how you navigated through your research journey.