Planning Your Data Collection: Designing methods for effective research
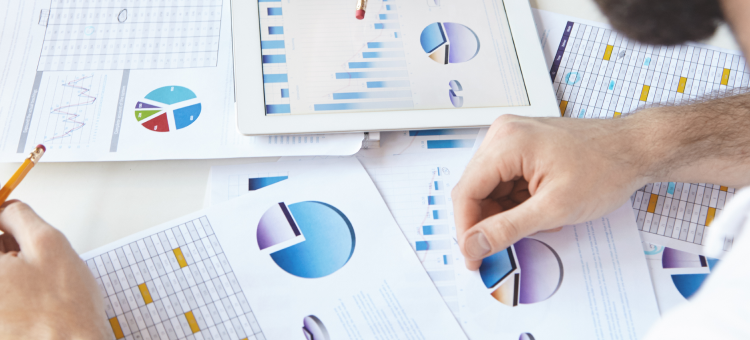
Planning your research is very important to obtain desirable results. In research, the relevance of data cannot be overlooked. It plays a pivotal role in laying a foundation to your study. Improper data has the potential to introduce bias and question the validity of your findings. Therefore, data collection is a critical step of any research project. It involves strategizing the process of gathering data to ensure its accuracy, and reliability. Without a thoughtful and systematic approach to data collection, researchers compromise the integrity and validity of their findings. By understanding the principles and strategies behind planning data collection, researchers and academicians can enhance the quality and impact of their research endeavors.
What Is Data Collection?
Data collection is the systematic gathering and measurement of information from relevant sources to address a research problem. It forms the backbone of any research, as it helps in decision-making and builds the foundation to establish solid conclusions. A data collection plan is an outline of the steps to gather data for research.
Purpose of Data Collection
The data collection element of research is common to all fields of study. Collecting data without a proper strategy can result in inconclusive or unreliable findings. To ensure the success of your research, it is essential to develop a comprehensive data collection plan.
Types of Data Collection Methods
The data collection process is approached by various methods that can be categorized into quantitative, qualitative, and mixed approaches. However, it is important to understand each method and select the most appropriate one to effectively achieve your research objectives.
9 Steps for Planning Your Data Collection
Planning your data collection is important to achieve directionality in your research. Following a proper plan can help you to simplify your data collection procedure as it organizes the entire process. The steps for planning your data collection are as follows:
1. Define Your Research Objectives
Before beginning data collection, it is essential to clearly formulate your research objectives. Defining these objectives will facilitate the identification of the types of data that need to be collected. Formulate research questions and try to define them. This will establish a clear direction for the study.
2. Identify the Data Requirements
After defining the research objectives, you must identify the specific data elements required to address the research questions. Furthermore, identify the available and accessible data and assess its efficacy. Consider both qualitative and quantitative data sources, such as surveys, interviews, observations, existing datasets, or experiments. Also, determine the level of detail for each data point and the suitable methods for data collection.
3. Select Appropriate Data Collection Methods
Choose data collection methods that align with your research objectives and data requirements. Evaluate the strengths and limitations of each methods and select the most appropriate approach accordingly. There are various data collection tools to choose from, such as interviews, role-playing, focus groups, in-person surveys, online surveys, telephonic surveys and observation. Assess the feasibility of the selected method and understand the pros and cons of each technique to make an informed decision.
4. Set a Realistic Timeline
Set a realistic timeline for your data collection process. This will not only help to organize your study but also ensures that you arrive at a conclusion within a specified timeframe. However, consider the time required for method design, pilot study, data interpretation, and analysis. Additionally, consider the available resources and constraints to create a feasible timeline.
5. Design the Method
After selecting your data collection process, design the method using the necessary instruments or resources. For surveys, create clear, concise, and unbiased questions that effectively capture the desired information. Develop interview protocols that cover the key topics you wish to explore. Carefully design observation protocols to ensure consistency and accuracy in data recording. Identify ways to collect maximum useful data and establish methods to interpret it. Also, determine ways to accurately measure the collected data.
6. Pilot Testing
Before launching your data collection, conduct a pilot test to evaluate the effectiveness of your instruments and procedures. A small-scale trial run allows you to identify any ambiguities in the data collection process. Also, make the necessary changes based on the pilot test feedback to enhance the reliability of your data.
7. Standardization
Establish a detailed standardized protocol based on the type of data and the results of the pilot testing. Also, record the specific instruments and standard conditions required for the study. Standardization of the protocol can facilitate the repetition of the study to check its reproducibility.
8. Establish Data Collection Procedures
Outline step-by-step procedures for data collection. Clearly document the process, including instructions for administering surveys, conducting interviews or observations, and handling any ethical considerations. Moreover, the researcher must be well-trained with the data collection method and must ensure its clear documentation.
9. Data Analysis Plan
Parallel to developing your data collection strategy, it is essential to plan your data analysis process. Determine and design the statistical methods or qualitative analysis techniques to derive meaningful insights from the collected data. Operationalize the data for variables that cannot be measured. Also, determine how to effectively represent your data.
Advantages and Disadvantages of Data Collection Methods
Understanding the advantages and disadvantages of the data collection method aids in planning your data collection method efficiently.
Following a structured plan for data collection can ease the process of research. Regardless of the field of the study, accurate and honest data collection plays a pivotal role in maintaining research integrity. Therefore, ethics should be at the forefront of any data collection strategy.
Ethical Reporting of the Collected Data — Especially with the dawn of AI
In the era of rapid technological advancements, the role of artificial intelligence (AI) in data collection and analysis has become increasingly prominent. Good research data management practices and principles of data sharing have become all the more crucial with the advent of AI in research data management. AI offers immense potential for processing and analyzing large datasets, enabling researchers to uncover valuable insights. However, using AI in data management accompanies several concerns.
While AI simplifies the hassles of data management, it must be handled following ethical principles. Therefore, it is crucial to address ethical considerations when reporting the collected data using AI to ensure responsible and transparent research practices.
Are you a researcher clueless of managing your research data and understanding the proper use of AI? Watch this webinar “Important tips for managing your research data” for FREE and get some clarity.
Six ways to ensure ethical reporting of collected data are:
1. Informed Consent
Obtaining informed consent from participants is an integral part of ethical principles in research. Therefore, participants must be adequately informed about the procedures, potential risks, and the expected duration of their commitment.
2. Data Privacy
Respecting participants’ rights to privacy is an important ethical guideline that researchers must adhere to. When using AI for data collection, participants should be informed about the involvement of AI algorithms and how their data will be used. Also, researchers should employ adequate measures to protect participants’ data, such as anonymization and de-identification techniques. Prior to reporting data, personal identifiers should be removed or masked to prevent the identification of individuals. Furthermore, employing different privacy techniques can protect participants’ identities.
3. Transparency
Transparency becomes crucial when utilizing AI algorithms for data analysis. Therefore, researchers should strive to provide clear explanations of the algorithms used and how they influence the analysis and reporting of data. This includes disclosing any biases, limitations, or potential errors associated with the AI algorithms used. Consequently, transparent reporting enhances the accountability of research findings.
4. Mitigating Bias
AI algorithms are prone to inheriting biases present in the training data. Researchers must be aware of these biases and evaluate them. Additionally, AI systems should be monitored for potential biases related as race, gender, or other sensitive attributes. Therefore, actions should be taken to introduce fairness and mitigate bias in the reporting of results.
5. Ensuring Data Security
Safeguarding collected data against unauthorized access or breaches is vital to ensure data security. Implement robust security measures to protect data integrity and confidentiality. Also, utilize encryption techniques, access controls, and secure storage protocols to prevent unauthorized access or data leaks. Clearly communicate these security measures in your reporting to assure participants that their data is handled responsibly.
6. Ethical Reporting of Results
When reporting findings, researchers must present data accurately and objectively. Avoid misrepresentation or manipulation of data to support predetermined conclusions. Also, acknowledge uncertainties or limitations associated with the data collection process and the involvement of AI. Clearly document and provide detailed descriptions of the data collection methods, AI algorithms used, and data analysis techniques employed.
By prioritizing ethical considerations when reporting data collected using AI, researchers can uphold the integrity of their research and ensure the protection of participants’ rights. Transparently documenting the data collection process, ensuring data privacy, addressing bias in AI algorithms, and maintaining accountability and reproducibility contribute to responsible and trustworthy reporting practices in the AI-driven research landscape.
Have you faced any challenges during data collection for your research? Share your experience in the form of a thought piece or an article on Enago Academy Open Platform.
Frequently Asked Questions
Data collection is important because it helps in making informed decisions and provides the answers to your research questions.
Data collection in research is a step where information is gathered based on variables of interest, in a systematic fashion that enables one to answer stated research questions, test hypotheses, and evaluate outcomes.
Data collection methods can be broadly classified as:
• Qualitative method: Qualitative methods deals with descriptive and conceptual data.
• Quantitative method: Quantitative method deals with numerical data which can be ranked or measured.
• Mixed method: Mixed method deals with both the numerical as well as the conceptual data.
Data can be collected for research using different means like interviews, surveys, observation, focus group discussion, forms, online monitoring, experiments, etc.